Focus on what’s not already regulated elsewhere!
As I write this, AI is cool and scary and full of potential. We’re in a race towards commercialization of the rudimentary (but powerful!) generative systems that machine learning has made possible. Artificial General Intelligence (AGI) – an AI system that is self aware and reasons like a human – is still a long way off, but our understanding of neuroscience is maturing rapidly and all indications are that the biological circuits that make us intelligent are built by massively scaling and interconnecting a small set of basic biological-algorithms and a vast array of sensory inputs.* AGI is a completely separate branch that really deserves a name of its own. Thinking about regulation of generalized AI completely under-scopes the seismic societal impact it will one day spawn. It will upend the world of ethics, philosophy, religion and what it means to be “a person.” I’m reasonably confident we’ll spot systems like that in advance, and at any rate that’s not what AI is now, so talking about regulating it is nonsense.
Regulating AI (the non general kind) is timely and worth pursuing.
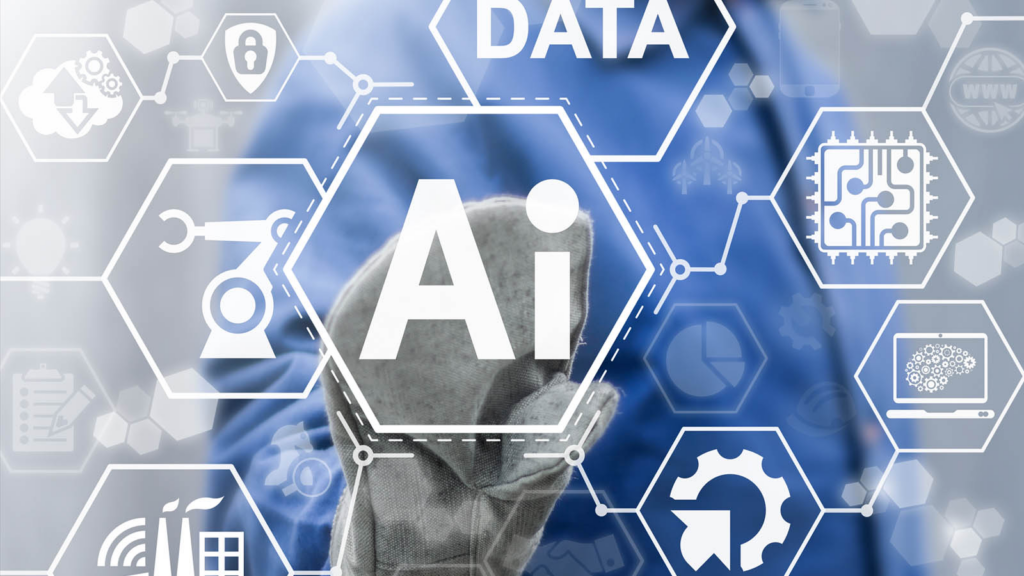
Let’s start with a characterization of AI that I think helps frame the discussion of the gaps AI regulation needs to fill. Today’s AI systems focus on data and the patterns that large datasets contain. They are extrapolation and interpolation engines that scale to problems both larger and more nuanced than what human brains can typically handle. They are not intelligent, or aware, but rather they are clever machines that produce results similar to what humans produce, but don’t process information in the way our brains do. Technologists use more precise terms like machine learning, or refer to specific AI techniques like large language models, but the umbrella term AI gets all the press. To understand how regulation applies to today’s AI, we need to focus on what makes AI unique from general computing and also from regulation of human systems that target the same tasks. (Pro tip: know the difference between AI, generalized AI, and machine learning.)
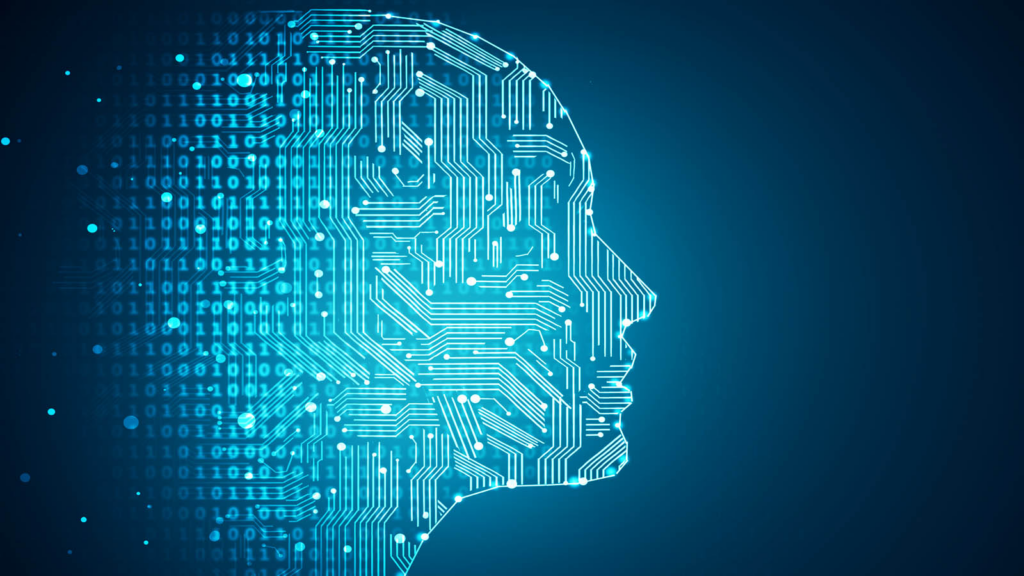
Let’s use autonomous vehicles as an example. Driving is already highly regulated. We verify a new driver’s training, eyesight, knowledge of the rules, safe driving techniques, etc. These rules evolved based on the risks and experience we’ve gained as a car culture, and an autonomous car should probably include all these safeguards with the AI regulated just like a driver. We also assume that today’s drivers have “common sense”, for example being aware that when a police officer sets a flare in front of your car you should probably take notice. Autonomous cars therefore need some additional regulations that capture the base assumptions we just assume safe drivers have, like obeying verbal commands from a uniformed officer. Another example might be workforce recruitment. HR professionals are typically experienced, aware and in compliance with anti-discrimination and employment laws, and have a sense for the limits of their expertise. For areas of the economy that already have laws and regulations governing the human systems involved, adding an addendum that focuses on AI in that specific domain is good regulatory practice. Regulation of AI systems should be an add-on to domain-specific regulation of the human systems that currently perform similar functions.
Treating AI as an efficiency or performance tool for specific domains solves most of the regulatory challenges this emerging technology brings. But AI as a computer system is also unique in its reliance on data. Data intensity is a characteristic of all AI systems, and thus deserves its own regulatory oversight (rather than repeat this in every domain where AI is later applied). Data regulation is an under-developed field in the U.S., but data privacy, data bias, data security and ethics are well developed in specific fields such as medicine and social science. The mechanisms already in use in domain-specific human systems – peer review, ethics panels, anonymization, etc. – are generally applicable to AI regulation. Data regulation is a co-requisite of AI regulation, and complements domain-specific AI regulations.
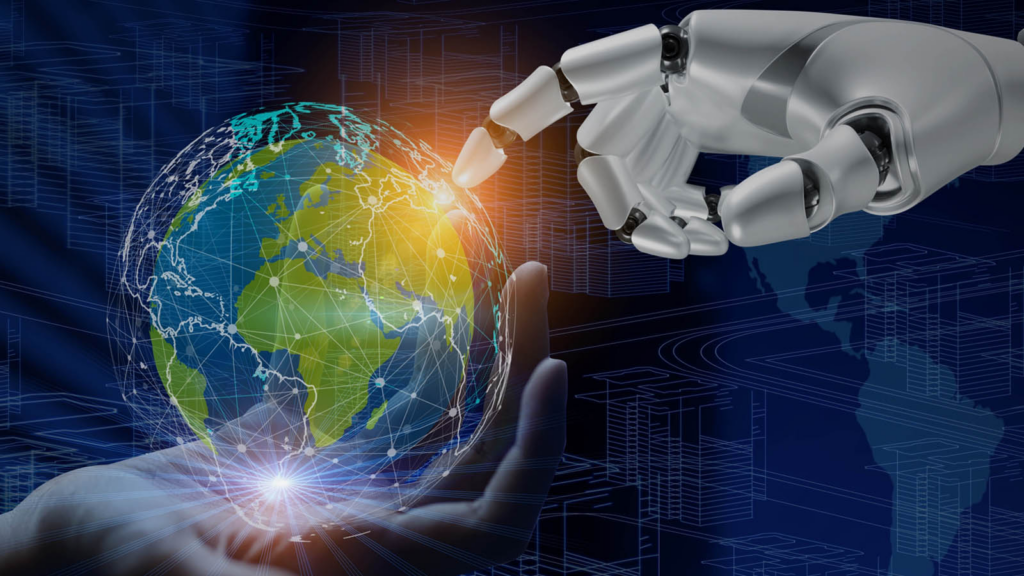
Finally, there is the disconnect between human systems and personal liability, and AI systems where liability is unclear. A general AI regulation should provide a bridge from existing common law and legal liabilities for legal persons, and re-attach it where an AI system is sitting in the person’s place. The law is well developed for when people or corporations cause harm, but what if the cause is AI acting in a person’s place? Fault in an autonomous car accident should be no more difficult to assign than in the current human-centric model. AI systems need to have an “owner” who retains liability for harms the system creates. This may entail obligations for systems with “known dangerous propensities” (shout out to Dedman law, class of 2008, who might still have nightmares over that assignment!).
Good AI regulation should be largely at the domain-specific level; adding specific AI requirements to existing domain regulation. At the aggregate level, regulation should focus solely on the characteristics that make AI unique; its reliance on data and the issues that creates, and its unsettling of the personal liability framework that underpins current law. Under no circumstances should AGI be incorporated into any of these frameworks, but the debate on artificial thinking beings is well worth pursuing far in advance of their arriving on the scene.
* Thanks to Jeff Hawkins for his insights in this area, and for sparking the idea that processing only the non-linear changes in otherwise linear or static systems is evolutionally efficient. I, for one, bow to my AI overlords. 🙂